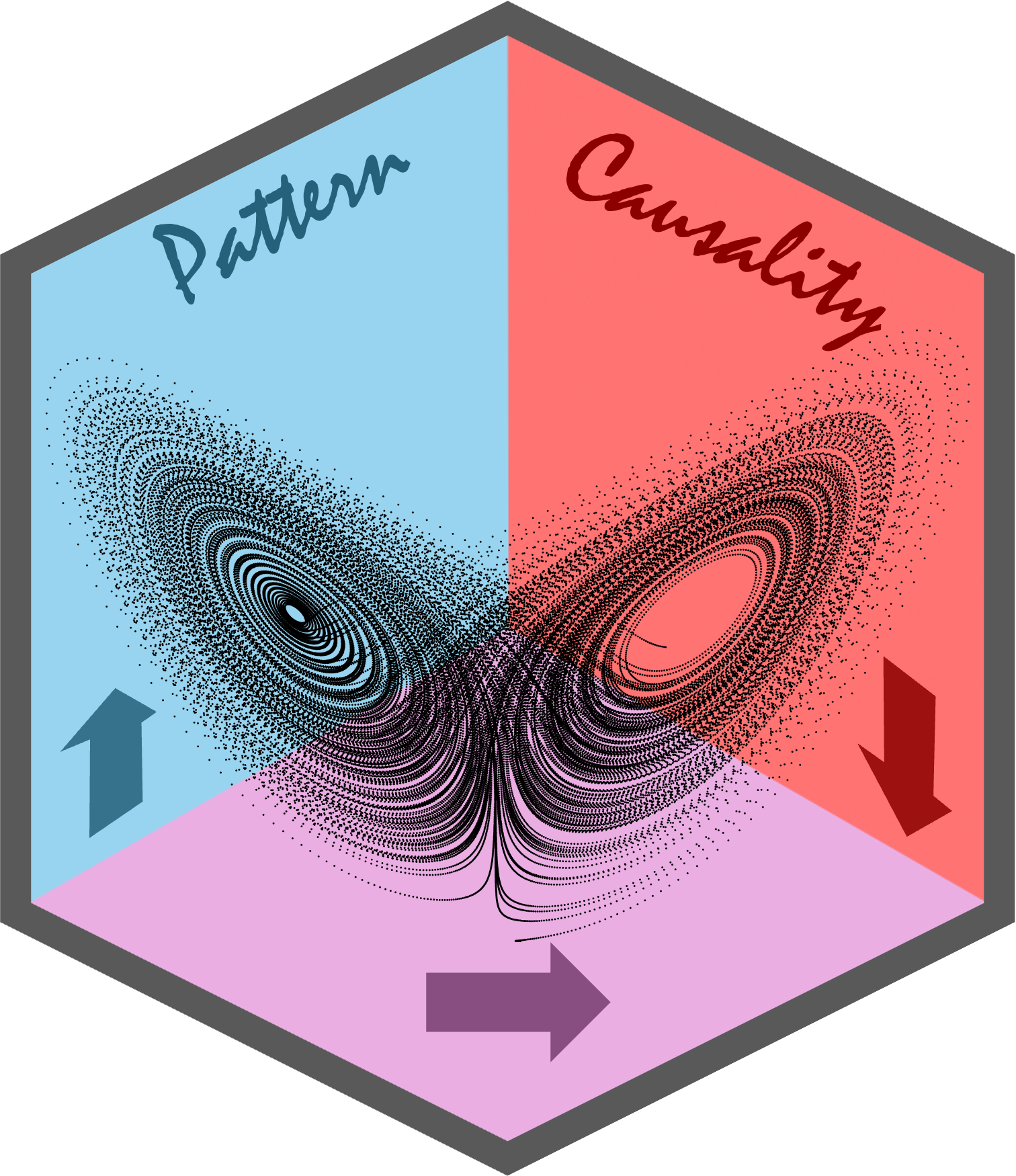
Parallel Computing in Pattern Causality Analysis
Stavros Stavroglou, Athanasios Pantelous, Hui Wang
Source:vignettes/parallel.Rmd
parallel.Rmd
Pattern causality analysis involves computationally intensive tasks,
especially when dealing with complex systems and large datasets. This
vignette demonstrates how to leverage parallel computing capabilities in
the patterncausality
package to significantly reduce
computation time and improve efficiency.
Key Benefits of Parallel Computing
The parallel computing features in this package are particularly effective for:
-
Bootstrap Analysis:
- Distributing bootstrap iterations across multiple cores
- Ideal for uncertainty quantification
- Significant speed improvements for large numbers of iterations
-
Matrix Computations:
- Processing large causality matrices efficiently
- Handling multiple time series simultaneously
- Reducing computation time for system-wide analyses
-
Cross-validation Studies:
- Parallel processing of different sample sizes
- Efficient handling of repeated computations
- Improved performance for robustness analysis
Performance Comparison: Sequential vs Parallel Computing
Let’s explore how parallel computing can enhance the performance of different pattern causality analyses:
library(patterncausality)
data(climate_indices)
Function to measure execution time
run_cv_test <- function(n_cores) {
start_time <- Sys.time()
result <- pcCrossValidation(
X = X,
Y = Y,
numberset = c(100, 200, 300, 400, 500),
E = 3,
tau = 2,
metric = "euclidean",
h = 1,
weighted = FALSE,
random = TRUE,
bootstrap = 100,
n_cores = n_cores,
verbose = TRUE
)
end_time <- Sys.time()
return(difftime(end_time, start_time, units = "secs"))
}
Compare sequential vs parallel
time_seq <- run_cv_test(1)
time_par <- run_cv_test(parallel::detectCores() - 1)
cat("Sequential computation time:", time_seq, "seconds\n")
cat("Parallel computation time:", time_par, "seconds\n")
cat("Speed-up factor:", as.numeric(time_seq) / as.numeric(time_par), "x\n")
Matrix Analysis with Multiple Time Series
When analyzing causality between multiple time series, parallel computing can significantly reduce computation time:
# Create larger test dataset
n_series <- 20
n_points <- 1000
test_data <- matrix(rnorm(n_series * n_points), ncol = n_series)
colnames(test_data) <- paste0("Series_", 1:n_series)
# Function to measure execution time
run_matrix_test <- function(n_cores) {
start_time <- Sys.time()
result <- pcMatrix(
dataset = test_data,
E = 3,
tau = 2,
metric = "euclidean",
h = 1,
weighted = FALSE,
n_cores = n_cores,
verbose = TRUE
)
end_time <- Sys.time()
return(difftime(end_time, start_time, units = "secs"))
}
# Compare sequential vs parallel
time_seq <- run_matrix_test(1)
time_par <- run_matrix_test(parallel::detectCores() - 1)
cat("Sequential computation time:", time_seq, "seconds\n")
cat("Parallel computation time:", time_par, "seconds\n")
cat("Speed-up factor:", as.numeric(time_seq) / as.numeric(time_par), "x\n")
Understanding Parallel Performance
Key Factors Affecting Speed-up
-
Data Characteristics
- Size of time series
- Number of series
- Sample sizes in cross-validation
- Number of bootstrap iterations
-
Hardware Considerations
- Number of CPU cores
- Available memory
- System architecture (Windows/Linux/Mac)
-
Analysis Type
- Bootstrap analysis: Excellent parallelization potential
- Matrix computation: Good for large matrices
- Cross-validation: Depends on sample sizes
Best Practices for Optimal Performance
# Get available cores
n_cores <- parallel::detectCores()
# Use n_cores - 1 for computation
recommended_cores <- max(1, n_cores - 1)
cat("Recommended number of cores:", recommended_cores, "\n")
# Example of memory-efficient parallel computation
result <- pcCrossValidation(
X = X,
Y = Y,
numberset = c(100, 200, 300),
E = 3,
tau = 2,
bootstrap = 50,
n_cores = 2, # Use modest number of cores for memory efficiency
verbose = TRUE
)
Conclusion
Parallel computing in pattern causality analysis can provide significant performance improvements, especially for: - Large-scale bootstrap analysis - Multi-series causality matrices - Extensive cross-validation studies
Choose parallel computing parameters based on: - Your system capabilities - Dataset characteristics - Analysis requirements - Available computational resources
For optimal results, always monitor system performance and adjust parameters accordingly.