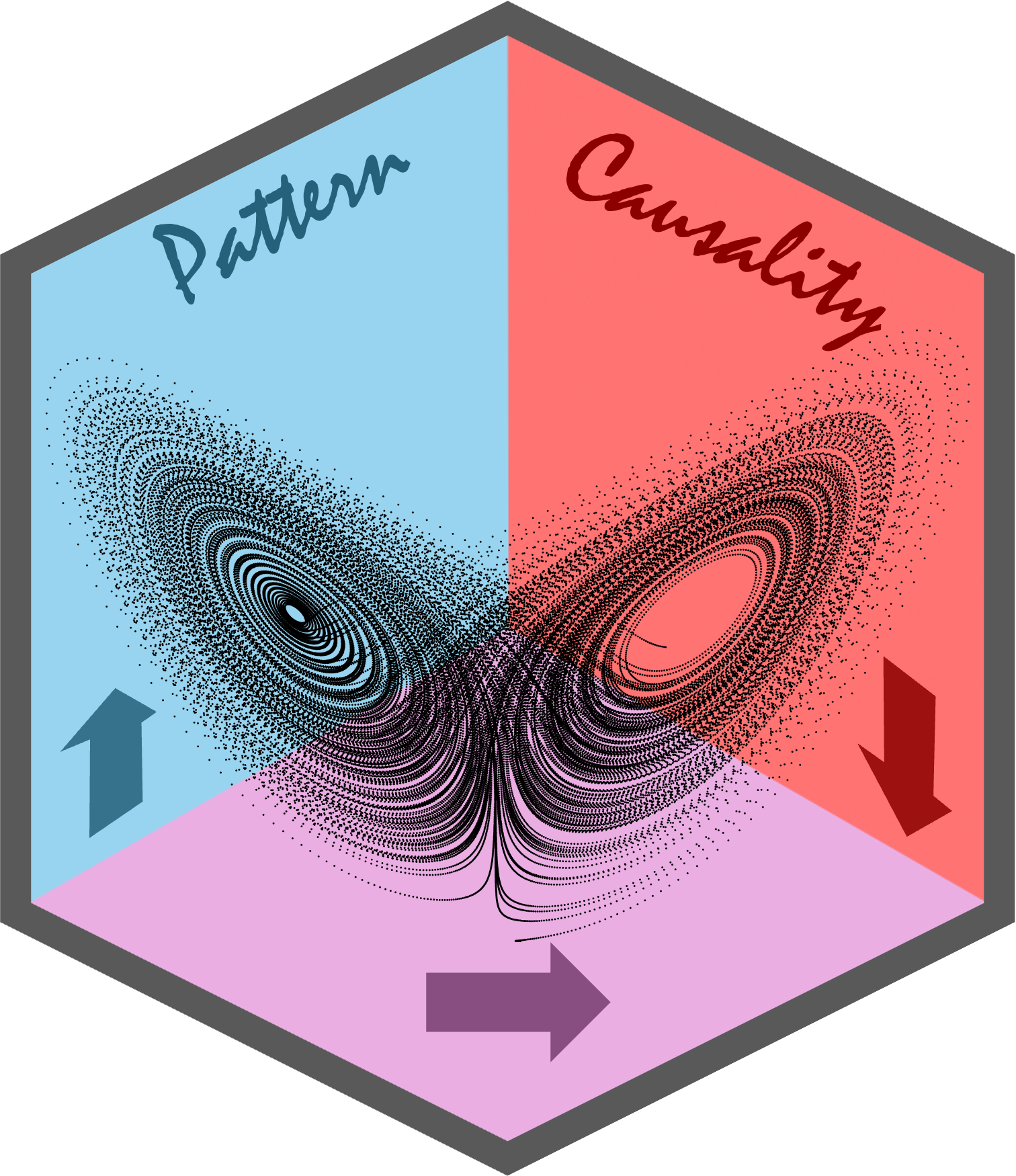
First Causality Point Check Function
Source:R/firstCausalityPointCHECK.R
firstCausalityPointCHECK.Rd
Checks if the time series data length is sufficient to perform causality analysis based on the provided embedding dimension, time delay, and prediction horizon. This function returns a Boolean indicating the feasibility of conducting the analysis.
Arguments
- E
An integer representing the embedding dimension, which influences the number of dimensions in which the time series is reconstructed for analysis.
- tau
An integer representing the time delay, used in reconstructing the time series in the embedded space. Note that in this version of the function, 'tau' is not actively used in calculations.
- h
An integer representing the prediction horizon, indicating how far ahead in the time series the predictions are aimed.
- X
A numeric vector representing the time series data.
Value
A boolean value; 'TRUE' if the time series is long enough to accommodate the specified parameters without running out of data, 'FALSE' otherwise.
Examples
time_series <- rnorm(1000) # Generate a random time series of 1000 points
embedding_dim <- 3 # Set embedding dimension
time_delay <- 2 # Set time delay (not used in current implementation)
pred_horizon <- 1 # Set prediction horizon
# Check if the first causality point can be considered
is_feasible <- firstCausalityPointCHECK(embedding_dim, time_delay, pred_horizon, time_series)
print(is_feasible)
#> [1] TRUE